Regression Model in App Marketing: A Complete Guide
Introduction
In an increasingly competitive advertising ecosystem, data analysis has become a critical skill for mobile marketers. Thanks to statistical regression models, it is now possible to predict a campaign’s results, identify the most effective levers, and optimize ad spend.
These models help transform raw data into actionable insights, making it easier to make informed decisions on key metrics such as install volume, acquisition cost, and user retention.
What Is a Regression Model?
Definition
A regression model is a statistical analysis tool that helps explain the relationship between a target (dependent) variable and one or more explanatory (independent) variables.
In app marketing, the target variable might be the number of installs, conversion rate, or ROAS, while explanatory variables could include media budget, distribution platform, targeting, seasonality, and more.
Example:
If you spend €10,000 on Meta Ads and generate 2,000 installs, a regression model can help you understand how that relationship evolves if you increase the budget or switch platforms.
Different Types of Regression Model
There are various types of regression models suited to different kinds of data and goals:
- Simple linear regression: Useful when the relationship between variables is proportional (e.g., installs increase linearly with budget).
- Multiple linear regression: Incorporates several independent variables (e.g., budget, platform, campaign type).
- Non-linear regression: Used when data doesn’t follow a straight-line pattern (e.g., performance saturation beyond a certain spend level).
- Logistic regression: Used for binary outcomes, such as whether a user converts or not.
- Regularized regressions (Ridge, Lasso): Useful for limiting overfitting in complex models with many variables.
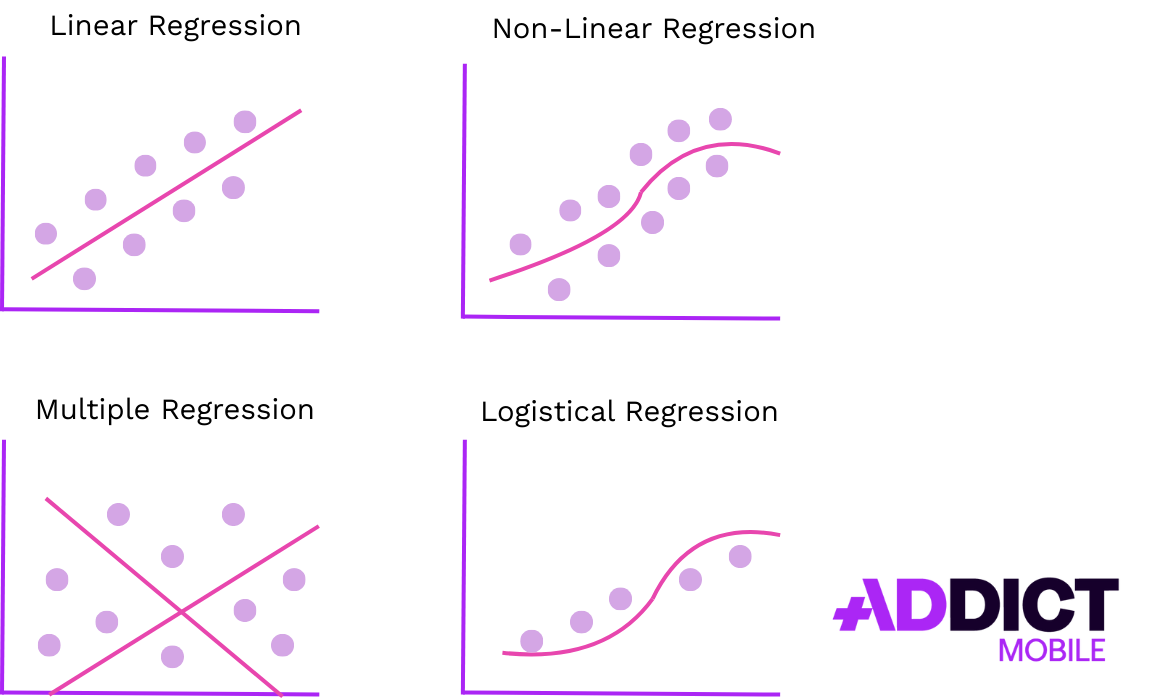
How It Works
Regression models are based on fitting a mathematical function to observed data. Typically, calculations aim to minimize the error between predicted and actual values. This error is commonly measured using:
- R²: Proportion of variance explained by the model.
- RMSE (Root Mean Square Error): Average prediction error.
- AIC / BIC: Indicators used to evaluate model quality.
Why Use Regression Models in App Marketing?
Regression models have been used in app marketing for over a decade, but widespread adoption began around 2015 with the rise of analytics tools, and surged post-2021 due to the loss of individual user tracking.
Regression models serve multiple key purposes in mobile marketing. Here’s why:
Predict Conversions Based on Advertising Spend
Regression models allow you to simulate the impact of changing budgets before launching a campaign. This helps forecast performance and guide investments toward the most effective channels.
Example:
Historical data may show that spending more than €15,000 on TikTok leads to plateaued conversions, whereas reinvesting in Meta still yields gains.
Optimize Campaign Profitability
By identifying which variables most influence performance, models help reallocate resources to the most profitable campaigns. They can also predict when a campaign hits a saturation point (diminishing returns on investment).
Example:
A multiple regression might show that campaigns running between 6–9 p.m. on Android deliver 25% higher ROAS than others.
Understand Performance Drivers
Regression models reveal which factors have the greatest influence on results (time of day, OS, channel, creative type, etc.), allowing marketers to prioritize optimizations.
Example:
You may find that iOS users aged 25–34 convert at twice the rate of other segments.
Data-Driven Decision Making
Regression brings mathematical and objective backing to decision-making—replacing guesswork and average-based assumptions.
Continuous Campaign Improvement
Models can be continuously updated with new campaign data to reflect current conditions and refine predictions.
Which Regression Model Should You Use?
Choosing the right model depends on several factors:
- The type of variable you’re predicting: continuous (e.g., spend, installs) or binary (e.g., conversion yes/no).
- The number and type of explanatory variables: a simple model might suffice if few variables are involved.
- The nature of the relationship: linear or non-linear.
- The size and quality of your dataset: the more data you have, the more advanced the model you can use.
👉 In app marketing, it’s common to start with a multiple linear regression, then move to more advanced models if needed.
Limitations of the Regression Model
While regression models are increasingly popular in mobile marketing, they do have limitations that can impact some advertisers’ results.
Requires Large Data Volumes
Regression models are only statistically reliable with sufficient data. A dataset that’s too small may yield unstable or unusable results.
Tip: Gather data over several campaigns and weeks before drawing conclusions.
Requires Data Over Extended Timeframes
Ad performance can vary by season, day of the week, or special events (sales, holidays…). An effective model needs to be trained on a dataset rich and representative enough to account for these factors.
Example:
A model based only on December campaigns likely won’t predict February performance well.
Needs Clear, Well-Defined Objectives
Before building a model, be clear about the question you want to answer:
- When is the best time to invest?
- Which channel drives the most profitable conversions?
- What ROAS can be expected at 30 days?
A poorly defined goal will lead to a poorly tuned model.
Best Practices for Implementing Regression Models
- Clean your data: Remove outliers, fill missing values, standardize formats.
- Check variable correlation: Some may be redundant or irrelevant.
- Test multiple models: Validate robustness using separate data sets.
- Visualize results: Use scatter plots, heatmaps, and curves for better interpretation.
- Iterate regularly: Models must be updated with fresh campaign data.
- Collaborate with data scientists: They can help fine-tune models and integrate them into your dashboards.
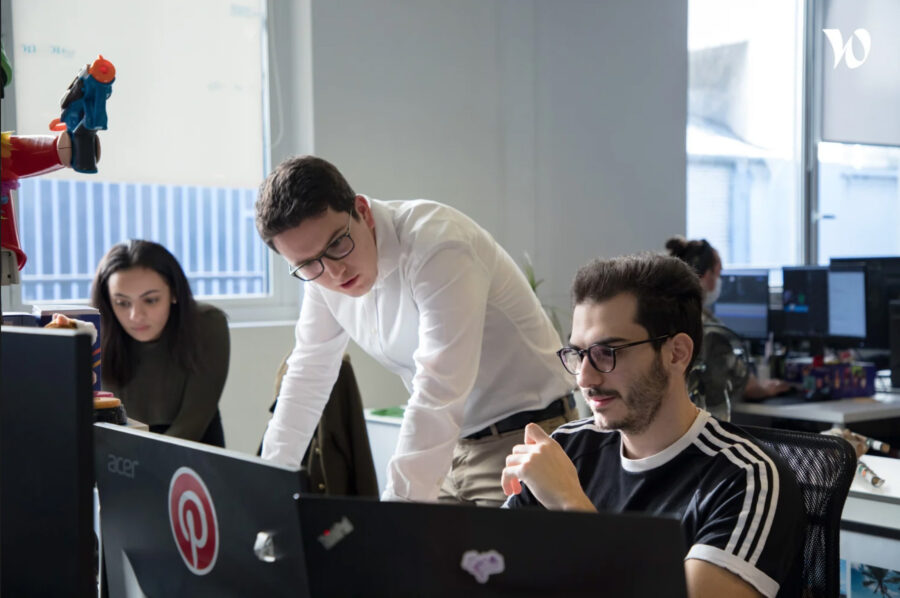
Do not hesitate to contact with our teams
Addict can support you to improve your performance.
Conclusion
Regression models are powerful allies for mobile marketers looking to make more accurate forecasts and improve campaign profitability. While they require a certain level of data maturity and solid foundations, their gradual implementation enables a shift toward more strategic, insight-driven campaign management—moving beyond intuition and guesswork.