Cohort Analysis: Definition, Examples, and Benefits in App Marketing
Introduction
In a mobile-first world where every user counts, deeply understanding audience behavior is key to optimizing marketing strategies. Cohort analysis has emerged as an essential tool to track performance trends over time. Whether you’re aiming to improve retention, fine-tune your campaigns, or make data-driven decisions, this method offers invaluable insights into your user base. Let’s break down what it is and how it can impact app marketing.
What Is Cohort Analysis?
Definition
A cohort is a group of users who share a common characteristic, usually tied to a specific point in time (e.g., their installation or signup date).
Cohort analysis involves tracking the behavior of that group over time to better understand how users engage with the app or respond to campaigns.
Example: Track the retention of users who installed the app during week 12 vs. those from week 13.
A Bit of History
Cohort analysis has been around for a while, but its rise happened in several stages:
- 2005–2010 – The Rise in SaaS and E-commerce: Cohort analysis began as a way to track customer retention and LTV, helping teams analyze behavior by user generation rather than through vague averages.
- 2010–2014 – The Mobile Explosion and User Tracking: App developers sought to understand early churn. This led to tools like Flurry, Mixpanel, and Appsflyer introducing simplified cohort views. Retention metrics such as D1, D7, and D30 became industry KPIs, and cohort-based retention became the foundation of mobile monetization funnels.
- 2015–2018 – In-App Monetization and Mobile Attribution: In-app revenue surged (+300% between 2014 and 2018, per Sensor Tower), pushing marketers to prioritize long-term user engagement. Attribution platforms like Appsflyer, Branch, and Adjust started including cohort views based on acquisition source—helping advertisers identify which channels bring the most valuable users. Cohort analysis became a key ROAS optimization lever.
- 2018–2022 – The AI Boom and LTV Prediction: Marketers could now collect and analyze massive data volumes, enabling LTV prediction from day 3 or 7 by combining cohort analysis with predictive modeling. Tools like Amplitude, Singular, and RevenueCat provided real-time cohort value tracking, allowing marketers to optimize bid strategies by cohort.
2021–Present – Post-IDFA & Privacy-First Era: With the end of individual tracking via IDFA, cohorts have become more essential than ever in app marketing. As data becomes less granular, users are grouped by behavior, acquisition source, or location. Cohort analysis is now a digital marketing standard.
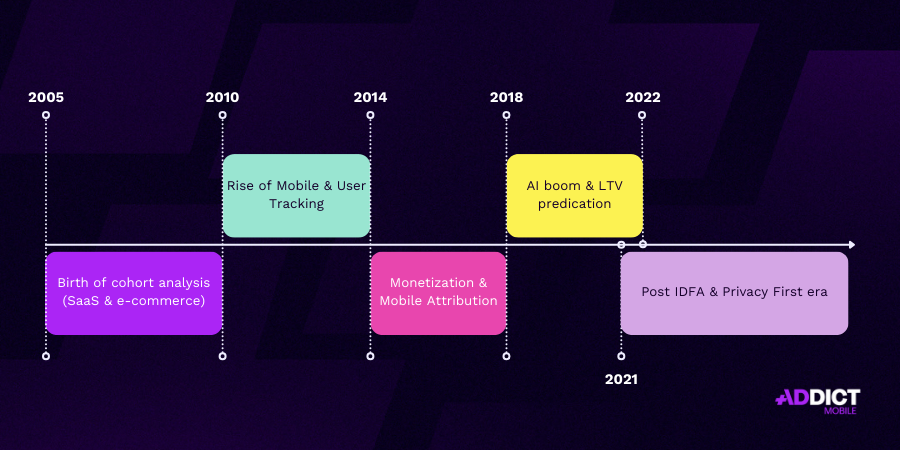
Different Types of Cohort Analysis
There are several approaches depending on your goals:
- User Acquisition Cohort Analysis: Segment users by acquisition source, channel, or campaign. This measures the quality of acquired traffic.
- Behavioral Cohort Analysis: Segment users by specific actions within the app (e.g., completing a tutorial or making a purchase). This reveals usage patterns and conversion dynamics.
Why Is Cohort Analysis Relevant in App Marketing?
In mobile marketing, data is everything. Cohort analysis provides a granular view of how user behavior evolves over time—rather than relying on flat averages. It empowers smarter decisions across several areas:
- Marketing: Understand the true profitability of a campaign beyond CPI.
- Sales: Feed LTV prediction models used for bid automation (Google UAC, Meta Ads).
- Product: Prioritize features and tests based on before/after cohort comparisons.
- Finance: It’s the most reliable way to justify budgets to a CFO or investor.
Benefits of Cohort Analysis in Mobile Marketing
Cohort analysis has become a standard because of its many advantages, such as:
Improved Customer Retention
By monitoring cohort retention, you can spot the drop-off points and take action to re-engage users—through better onboarding, personalized offers, or targeted notifications.
Better Understanding of User Behavior
Cohorts help visualize how users interact with your app after a key event, making it easier to optimize the journey and boost engagement.
Campaign Optimization
Instead of focusing only on CPI or volume, cohort analysis helps pinpoint which campaigns deliver the most long-term value (ROAS, LTV…).
Smarter Decision-Making
A clear cohort view ensures that decisions—whether in marketing, product, or CRM—are driven by real data, not gut feelings.
What Metrics Should You Track in Cohort Analysis?
The most common cohort metrics include:
- Retention rate (D1, D7, D30, etc.)
- Average sessions per user
- Lifetime Value (LTV)
- Conversion rate (signup, purchase, subscription)
- Churn rate
- ARPU (Average Revenue Per User)
These indicators help evaluate each cohort’s performance and identify optimization levers.
How to Use Cohort Analysis to Boost Revenue
Cohort analysis isn’t just for understanding—it’s a tool for action. Here are a few concrete examples:
Optimize Onboarding and Activation
By analyzing D1, D7, and D30 retention through install cohorts, you can assess the onboarding quality and make UX adjustments. A well-crafted onboarding flow increases the likelihood of return visits—and spending. According to a 2023 Appsflyer report, a 5% increase in D1 retention can lead to up to 85% higher long-term revenue. Apps with D30 retention over 20% are typically in the market’s top 25%.
Understand Paying User Life Cycles
Cohorts of users who made a first purchase allow you to track LTV and identify the behaviors that lead to conversions—then replicate or accelerate those behaviors in other cohorts.
Test and Compare UA Campaigns or A/B Tests
Create cohorts by acquisition source to reveal differences in retention or LTV. This shows which acquisition channels are truly profitable—not just which bring volume. In some verticals, organic users drive 30% higher LTV than paid ones (Source: Appsflyer – 2024 Performance Index).
Monitor the Impact of New Features or Updates
Track pre- and post-release cohorts (e.g., for premium features or social tools) to see which updates positively affect revenue.
Forecast Monetization With LTV Patterns
Cohort analysis lets you model LTV growth over 60 or 90 days. You can then forecast expected ROAS and adjust UA budgets accordingly. Apps with solid LTV modeling generate 25% more paid acquisitions on average (Source : Singular).
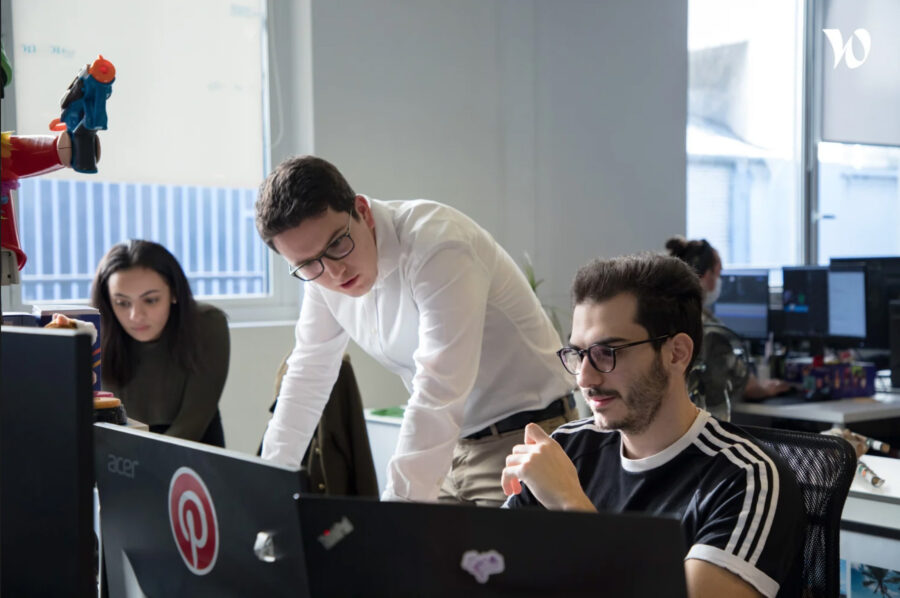
Do not hesitate to contact with our teams
Addict can support you to improve your performance.
6-Step Methodology for Cohort Analysis
Here’s a simple checklist of the 6 key steps to run a successful cohort analysis:
1. Define the Cohort
Start by identifying a group of users who share a common point in time (first purchase date, install date, level reached, ARPU > X€…). Weekly or monthly grouping is ideal, depending on your user volume.
2. Choose a Metric to Analyze
Select a key behavior to track over time—such as retention (D1, D7, D30), revenue (ARPU, cumulative LTV), conversion rate, sessions, etc.
3. Track Changes Over Time
Once your metric is selected, assess how it evolves post-event. Create a matrix where rows represent cohorts and columns represent days/weeks after the reference event. Use heatmaps to highlight patterns and anomalies.
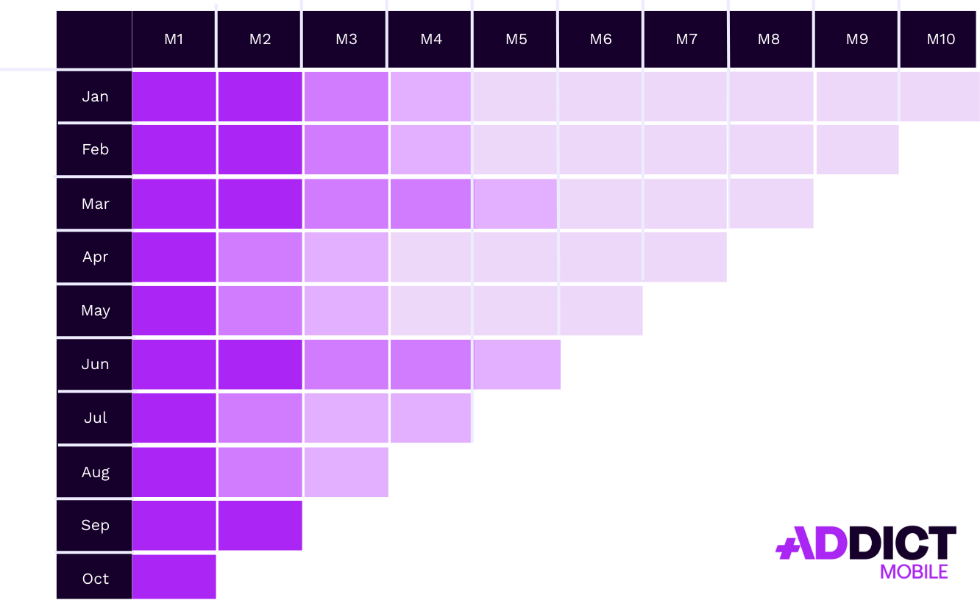
4. Segment Cohorts Intelligently
Once your matrix reveals behavioral differences, segment groups by acquisition source (TikTok, UAC, organic…), geo-location, device, OS, etc. This allows deeper insight into performance drivers.
5. Identify Patterns and Insights
Now that you have segmented cohorts, you can detect which ones have the highest LTV, which channels yield positive effects, or where churn most frequently occurs in the user journey.
6. Take Action
Use your insights to optimize acquisition, product design, and monetization. Invest more in top-performing sources, adjust onboarding flows, highlight key features, or adapt offers to the user lifecycle.
Recommended Tools
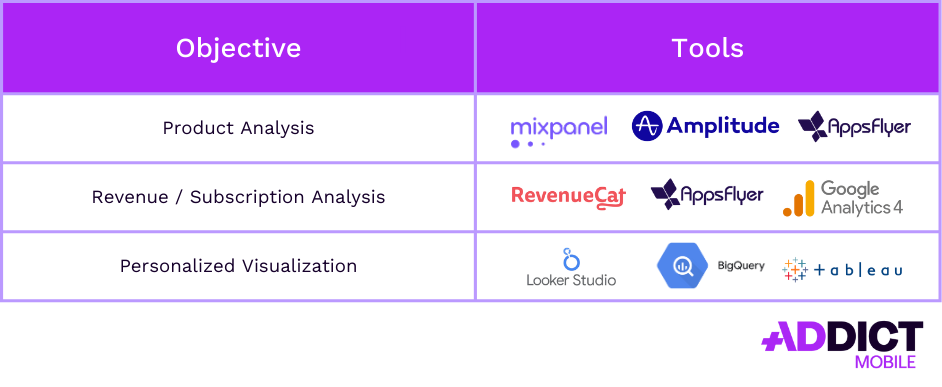
Conclusion
Cohort analysis is a must-have technique in app marketing to drive revenue and ensure product-market fit. By following a 6-step approach, you can unlock critical insights and perform the necessary optimizations to boost overall app performance.