Incrementality in Mobile Marketing: Definition, Methods, and Best Practices
Introduction
Incrementality is a key concept in mobile marketing, enabling the measurement of the real impact of a marketing action on an app’s performance. Unlike traditional attribution, which follows a deterministic model, incrementality seeks to answer a crucial question: “What portion of conversions would have happened even without the marketing action?”
In this article, we will explore how to measure incrementality, why it is essential in app marketing, and which methods allow for a detailed evaluation.
What Is Incrementality?
Definition
Incrementality is a metric that measures the additional impact of a marketing campaign. It determines the difference between the behavior of users exposed to an ad campaign and those who were not. The goal is to isolate the direct effect of the campaign and exclude conversions that would have happened naturally.
This concept is essential for understanding whether a marketing campaign has generated new conversions or merely captured users who would have installed the app anyway.
Calculation Method
The classic approach to calculating this value relies on comparing a test group (exposed to the campaign) with a control group (not exposed). The basic formula is as follows:
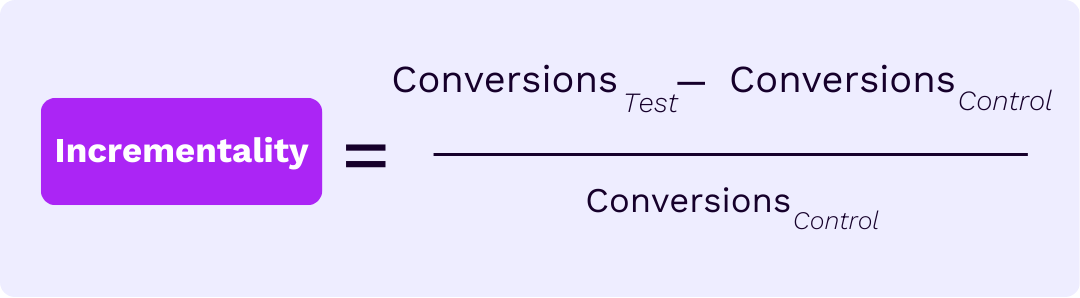
Other methods include more advanced analyses such as Bayesian models or machine learning algorithms to predict the impact of a campaign.
Why Measure Incrementality in App Marketing?
The Benefits
Understanding the Impact of a Marketing Action
Without incremental analysis, it is difficult to determine whether an advertising campaign truly influenced user behavior. For example, would a user who installs an app after seeing an ad have installed it anyway without that ad?
Proving the Business Value of a Channel or Action
Some campaigns appear successful in terms of conversion volume but do not actually provide incremental value. Measuring this value ensures that every dollar spent on advertising generates real added value.
Optimizing the Commercial Offer
Incremental analysis allows businesses to adjust their offers and focus on the most effective channels while eliminating unnecessary expenses.
Enhancing Marketing Mix Modeling
Traditional attribution models no longer provide a complete view of marketing impact. Integrating incremental analysis offers a more accurate perspective on campaign effectiveness.
Why Is It Crucial in App Marketing?
With the end of IDFA and increasing data privacy restrictions, directly attributing a conversion to an advertising source is becoming more difficult. This analysis provides a reliable alternative for measuring campaign effectiveness without relying on individual data.
How to Conduct Incrementality Analyses?
Several methods exist for conducting incremental analyses. The four main approaches are:
Causal Inference
Causal analysis uses advanced statistical techniques such as regression, Bayesian models, and randomization tests to establish cause-and-effect relationships.
A/B Testing
A/B tests allow for a direct comparison of a campaign’s effect by exposing one group while leaving another unexposed.
Geo-lifting
Geo-lifting tests a campaign’s impact by activating it in certain geographic areas and comparing results with other unexposed regions.
Budget Holdout
Temporarily pausing the advertising budget on a channel helps observe whether conversions drop or remain stable, indicating whether the channel is truly incremental.
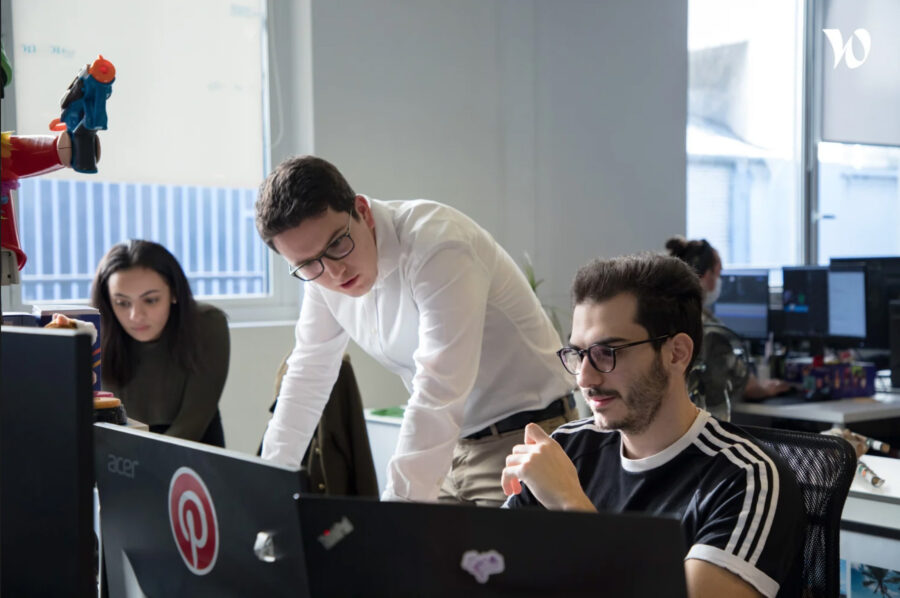
Do not hesitate to contact with our teams
Addict can support you to improve your performance.
Attribution vs Incrementality
Fundamental Differences
Attribution and incrementality are two complementary approaches to measuring marketing campaign impact.
- Attribution: Assigns a conversion to a marketing touchpoint (click, impression) based on a predefined model (last click, first click, multi-touch, etc.).
- Incrementality: Seeks to isolate the real impact of a campaign by comparing an exposed group to a control group.
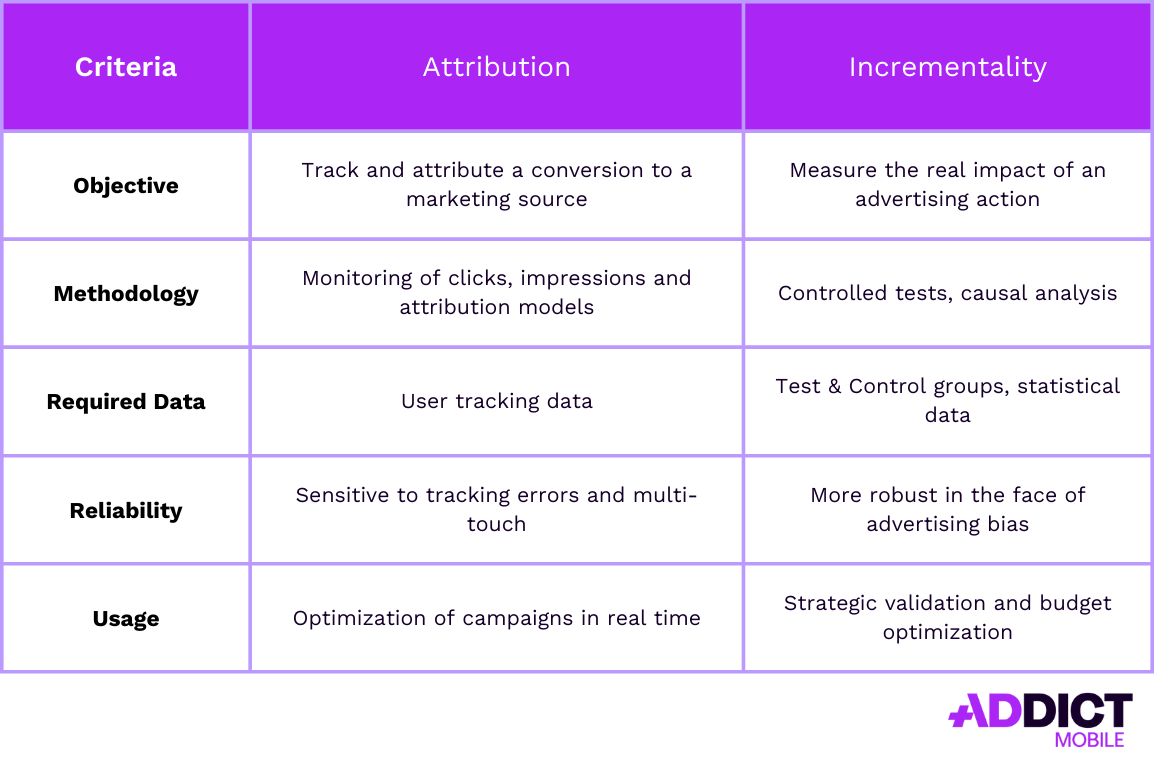
Complementarity
Attribution is useful for tracking campaign performance on a daily basis, while incrementality provides a broader view of their actual effectiveness. Both approaches should be used together to maximize advertising investment profitability.
Marketing Mix Modeling vs. Incrementality
Definition of Marketing Mix Modeling
Marketing Mix Modeling (MMM) is a statistical method that analyzes the effect of different marketing channels (TV, digital, offline) on sales or app installs. It uses econometric models to determine the contribution of each channel.
Differences with Incrementality
MMM focuses on high-level budget allocation and strategic decisions, while incrementality measures the short-term impact of specific actions.
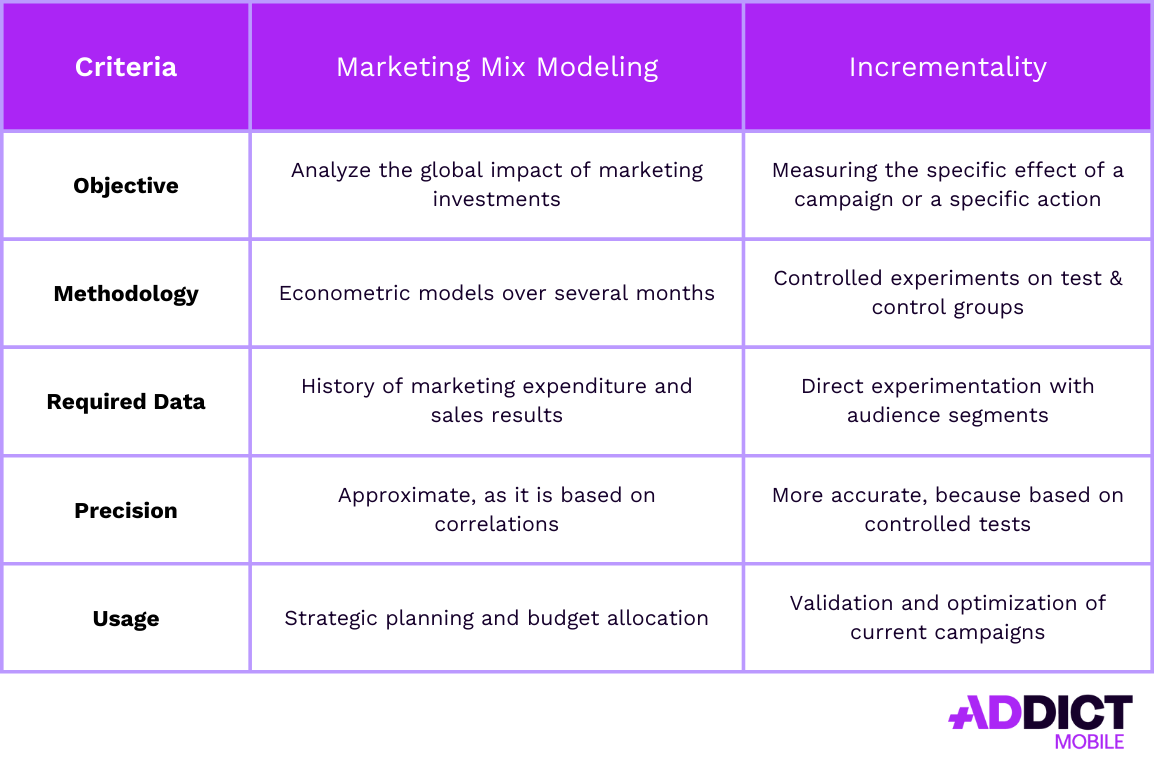
Quand utiliser l’un ou l’autre ?
- MMM is useful for macro decisions (annual budgeting, allocation between TV/digital channels).
- Incrementality is preferable for testing specific actions and measuring their real short-term impact.
By combining both, a company can gain a comprehensive view of marketing effectiveness and adjust investments accordingly.
Challenges Related to Incrementality
Despite its importance in marketing and attribution, several challenges can make incrementality analyses complex:
Difficulty in Isolating Marketing Effects
Incrementality requires distinguishing conversions attributable to a campaign from those that would have occurred naturally (organic effect). Several factors complicate this distinction:
- Existing brand awareness
- Consumers’ habitual purchasing behavior
- Influence of other marketing channels (multichannel effect)
💡 Solution: Use “holdout” tests (exposed vs. non-exposed groups) to observe performance differences.
Complexity of Tests and Methodologies
Incrementality analysis relies on rigorous methodologies such as:
- A/B Testing or Holdout Tests: Comparing an exposed group with a non-exposed control group.
- Advanced Statistical Models: Econometric modeling, causal inference, etc.
- Quasi-Experimental Approaches: Methods like “Synthetic Control” or “Difference-in-Differences.”
💡 Solution: Invest in tools and data science experts to ensure reliable analysis.
Attribution Bias
Traditional attribution models (last-click, first-click, linear, etc.) do not account for incrementality. They assign conversions without distinguishing those that would have occurred naturally from those truly generated by the campaign.
💡 Solution: Favor incrementality-based attribution models, such as:
- Geo-Experiments (regional testing)
- Uplift Modeling (predicting users influenced by the campaign)
Duration and Cost of Tests
These tests require time to collect a sufficient data volume, and implementation can be costly. Challenges include:
- Budget impact due to temporary campaign suspension for some groups (holdout).
- The need for a large number of conversions to achieve statistically significant results.
💡 Solution: Use hybrid methods combining direct tests and statistical modeling to accelerate results.
Influence of External Factors
Incrementality analysis can be biased by external events influencing consumer behavior independently of marketing:
- Seasonality (e.g., Black Friday, Christmas)
- Competition and rival promotions
- Economic fluctuations (inflation, crisis)
💡 Solution: Integrate control variables and adjust models to account for these factors.
Dependence on Advertising Platforms
Some platforms (Google, Facebook, etc.) offer incrementality measurement tools, but they have a vested interest in optimizing their own results, raising transparency concerns.
💡 Solution:
- Cross-reference data with independent tests.
- Use third-party incrementality analysis tools (e.g., Nielsen, Google’s CausalImpact).
Limited Applicability by Channel
Incrementality is easier to measure on digital channels with precise tracking (Facebook Ads, Google Ads) than on offline media (TV, billboards, radio), where direct exposure-to-conversion links are harder to establish.
💡 Solution: Adopt mixed approaches, combining statistical models and field experiments.
Conclusion
Measuring incrementality is essential for optimizing advertising investments, but it involves technical, methodological, and organizational challenges. The key is to adopt a rigorous approach, combining experimental tests and advanced modeling while integrating independent validation mechanisms.